The Food and Drug Administration grappled with questions about how to regulate generative artificial intelligence in medical devices at its first digital health advisory committee meeting.
To date, the agency has authorized nearly 1,000 AI-enabled medical devices, but none of those devices use adaptive or generative AI. However, the technology is being explored in other healthcare applications not regulated by the FDA, such as generating clinical notes for physicians.
“For us to be most effective in our jobs as protectors of public health, it’s essential that we embrace these groundbreaking technologies, not only to keep pace with the industries we regulate but also to use regulatory channels and oversight to improve the chance that they will be applied effectively, consistently and fairly,” FDA Commissioner Robert Califf said at the November meeting.
Generative AI can mimic input data to create text, images, video and other content. The technology poses unique challenges; models are often developed on such large datasets that developers may not know everything about them. Generative AI models also may change rapidly over time and can generate false content to meet a user’s prompt, known as “hallucinations.”
Experts met on Nov. 20 and 21 to discuss how the FDA can regulate this new technology and set a framework for safe and effective use of generative AI in healthcare.
Here are four takeaways from their discussion.
1. Patients want to know when AI is used
When Grace Cordovano, founder of the patient advocacy group Enlightening Results, received her latest mammogram results, they mentioned that “enhanced breast cancer detection software” had been applied.
Although the results were normal, the test picked up a number of benign findings. Cordovano called the imaging center because she wanted to learn where these findings were coming from, or if she could get a copy of the mammogram without the AI applied to compare them.
“I got a ‘ma’am, we don’t do that,’” Cordovano told the advisory committee. “A month later, I got a confusing letter saying, based on your mammogram, you now need to go for an MRI. So there’s just discrepancies, and I care, and I’m digging in.”
Cordovano said the majority of patients, about 91% according to a recent survey, want to be informed if AI is used in their care decisions or communications. Patients should also be recognized as the end users of generative AI-enabled medical devices and must have the opportunity to provide structured feedback when it’s applied to their care.
“I think it’s wonderful that the patient voice is being included, but we’re at a complete disadvantage here,” Cordovano said. “We have no idea where [AI is] being applied in our care. We don’t know at what point who’s doing it.”
The FDA’s digital health advisory committee agreed it may be important for patients and providers to know when they are using a generative AI-enabled device. The panel also recommended that patients be told how such a device contributed to their care and what information the device used in its decision-making.
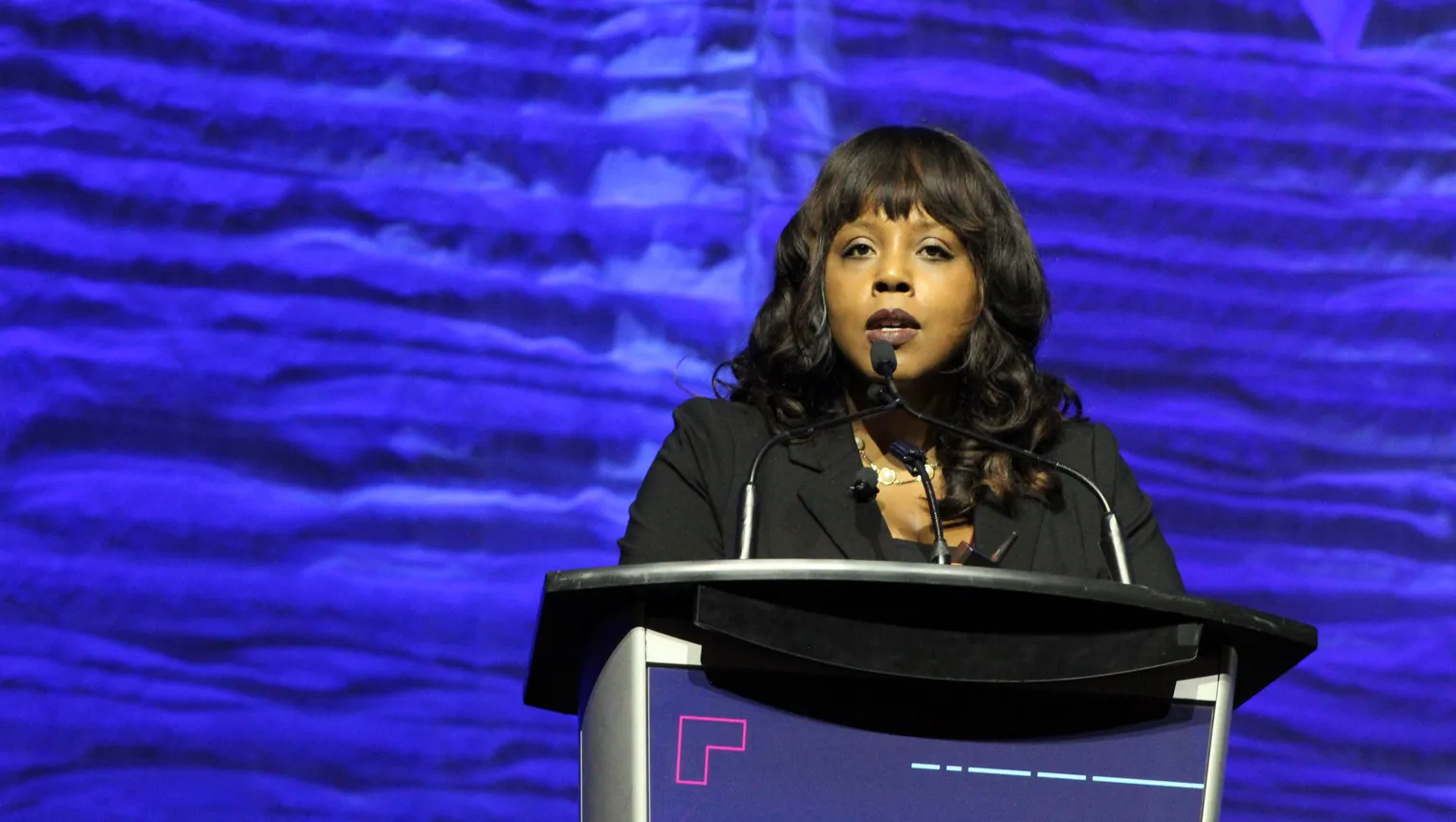
Michelle Tarver, director of the FDA’s Center for Devices and Radiological Health, spoke at Advamed’s The Medtech Conference on Oct. 17, 2024.
Elise Reuter/MedTech Dive
2. Health equity is at the heart of the debate around generative AI
Michelle Tarver, director of the FDA’s Center for Devices and Radiological Health, discussed the promise of AI in extending care to people in communities with fewer resources — people who are older, are racial and ethnic minorities, and who live in small towns further away from healthcare facilities.
However, that promise is matched by concerns the technology could amplify existing health inequities.
“This is really a collective conversation on how do we move things forward in an equitable and ethical way, and that requires including everyone at the table,” Tarver said.
Jessica Jackson, an advisory committee member and the founder and CEO of Therapy is for Everyone, said equitable device performance should be a metric, not just a nice-to-have.
“Historically, we have not been equitable in including data from marginalized communities in clinical trials and we have felt that that was still good enough,” Jackson said. “That needs to change for gen AI because we will be training future models on the data that is coming from these.”
Currently, the FDA requires manufacturers to share a review of their AI-enabled devices’ overall safety and effectiveness as part of the premarket review process, including an evaluation of study diversity based on the device’s intended use and characteristics. Sonja Fulmer, deputy director of the FDA’s Digital Health Center of Excellence, said the agency has denied products based on inadequate representation in performance data.
Jackson said the agency should spell out requirements that AI-enabled devices perform equitably postmarket across different communities.
“Clearly articulating that this needs to be considered in the postmarketing and premarketing stage is important,” said Chevon Rariy, a member of the advisory committee and chief health officer of Oncology Care Partners.
Rariy suggested that the FDA might not dictate exactly how manufacturers should monitor for equity and bias for a specific device, but it could recommend that such a plan exists.
3. Hospitals are still developing best practices for generative AI
Hospital representatives at the meeting outlined their frameworks for evaluating AI, but they also cautioned that facilities aren’t ready to use the technology unsupervised.
“I have looked far and wide. I do not believe there’s a single health system in the United States that’s capable of validating an AI algorithm that’s put into place in a clinical care system,” Califf said.
Michael Schlosser, HCA Healthcare’s senior vice president of care transformation and innovation, said the for-profit hospital system has developed a framework for evaluating and implementing AI. Anyone who uses AI in patient care must go through training.
Schlosser added that the organization is not ready to put a patient’s treatment, diagnosis or other aspects of care as the end result of a generative AI model.
“We’re very much tiptoeing into the use of generative AI,” Schlosser said. “We still have an enormous amount to learn from these models and how they perform, and why they do the things that they do.”
“I have looked far and wide. I do not believe there’s a single health system in the United States that’s capable of validating an AI algorithm that’s put into place in a clinical care system.”

Robert Califf
FDA Commissioner
Most AI devices used today are in radiology. These devices are fixed and don’t use generative AI. Keith Dreyer, chief data science officer at Mass General Brigham, said the technology is acting on data that’s coming out of a device, such as a CT scanner, MRI machine or EKG machine. But those devices, and the data coming out of them, are constantly changing.
“We have 2,000 scanners at our facility, and none of them are the same,” Dreyer said. “The concept of trying to have one device work on every single scanner … is just not realistic.”
He said that validation has to take place, especially for generative AI.
The American Medical Association also recommended that the FDA institute ongoing validation requirements to ensure AI tools perform safely and equitably. Frederick Chen, the AMA’s chief health and science officer, said more than a third of doctors use AI. Chen said the organization is disappointed that it has not yet seen transparency mandates from the FDA for AI-enabled medical devices.
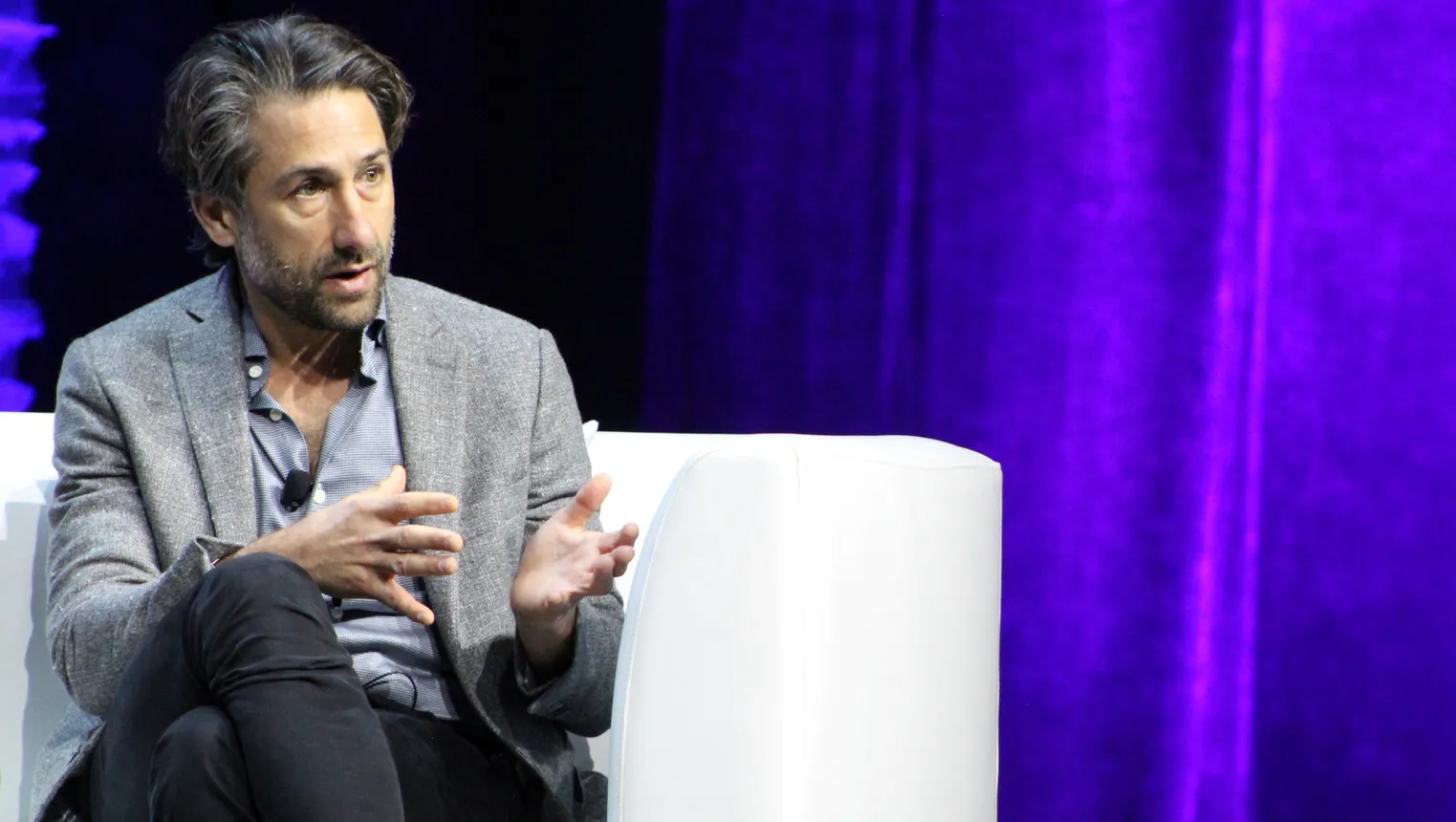
Troy Tazbaz, director of the FDA’s Digital Health Center of Excellence, spoke at Advamed’s The Medtech Conference on Oct. 17, 2024.
Elise Reuter/MedTech Dive
4. How to build a process for spotting, reporting errors
Generative AI models pose new regulatory challenges due to their nature.
“Unlike traditional AI models that predict or classify data, Gen AI produces outputs, which can introduce additional layers of regulatory complexity,” Troy Tazbaz, director of the FDA’s Digital Health Center of Excellence, said during the event.
One area of focus for the panelists was the challenge of identifying errors and setting boundaries for generative AI models.
Nina Kottler, associate chief medical officer for clinical AI at Radiology Partners, said the practice has used a generative AI tool that reads radiology reports and generates a summary of findings under the “impression” section. A radiologist will check and edit that section before sending off the report.
Although the tool is not regulated as a medical device, Radiology Partners evaluated the technology by having a panel of radiologists look at 3,000 reports, as well as comparing the AI-generated impression to the final, edited version. The AI-generated impression had a clinically significant error rate of 4.8%, while the final result edited by a radiologist had a 1% error rate.
“It’s very hard to go to a radiologist and say, just be careful on every single exam because 5% of the time it’s going to make a mistake.”

Nina Kottler
Associate chief medical officer for clinical AI at Radiology Partners
Kottler emphasized the importance of expert review and training so that people are aware of the types of errors generative AI is prone to making.
“It’s very hard to go to a radiologist and say just be careful on every single exam because 5% of the time it’s going to make a mistake,” Kottler said.
For example, generative AI can incorrectly infer things based on patterns. In one example, a radiology report talked about a femur bone but didn’t specify which leg. The AI model “guessed” the right femur but the report was actually about the left femur.
It can also make recommendations that aren’t appropriate, such as a follow-up MRI scan for a benign finding.
“Our validation on this AI model suggests it should not be autonomous,” Kottler said.
Oncology Care Partners’ Rariy said the FDA could play a role in defining adverse events and errors under generative AI and ensuring companies have a process in place if they determine additional “guardrails” are needed around a model.
“Perhaps as the FDA we could articulate a need to have a process in place,” Rariy said. “What is that device manufacturer’s process that they’re going to monitor and adhere [to] for various errors that come up?”
Rariy added that the process should include a clear reporting mechanism for patients and users if an error is found.