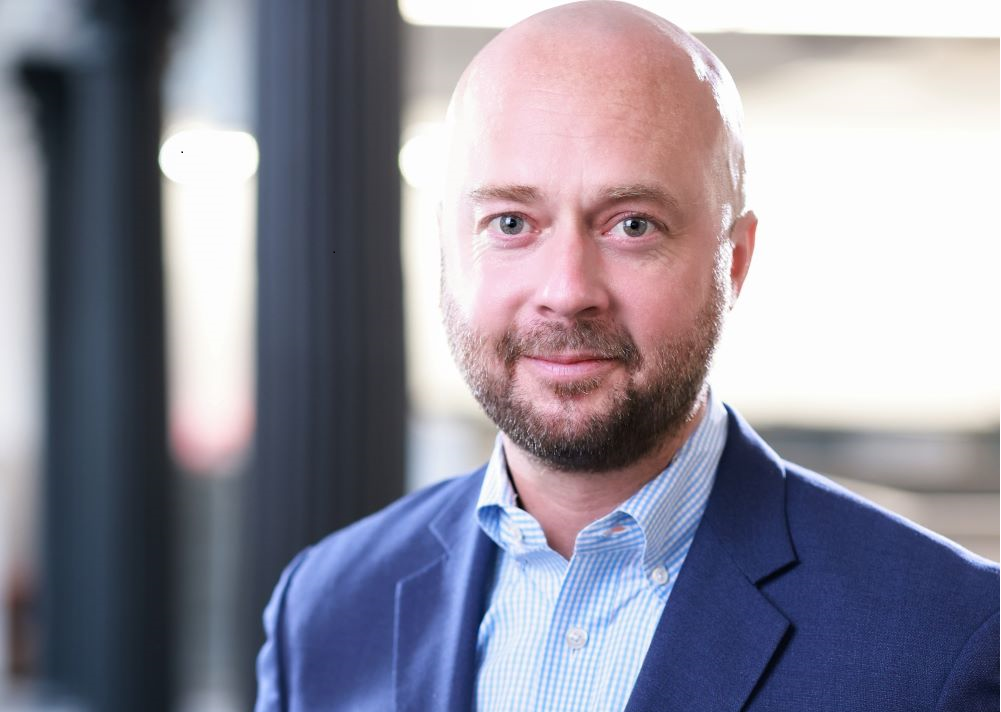
Data has become the lifeblood of healthcare, making it possible to gain granular insights that enable more effective and precise patient-centric care. Yet, we have just scratched the surface of data analytics’ potential impact on optimizing segments of healthcare that need it most, such as the surging arena of consumer health.
Of the four key types of data analytics – descriptive, diagnostic, predictive, and prescriptive – only the first two are effectively used in managing healthcare costs and member healthcare delivery. These two capabilities inform stakeholders about what has happened and why it happened. What might happen in the future, and determining the best course of action to take is often hard to assess at scale. Enter predictive and prescriptive analytics, which employ machine learning to analyze billions of possible scenarios and identify the optimal next step. Stakeholders today face an overwhelming amount of information to base decisions off of, but predictive and prescriptive analytics eliminate choice overload and prescribe the most impactful way forward.
Leveraging AI models to manage plan costs, identify potential emerging risks and high-cost claimants requires a proactive approach that focuses on prevention, early intervention, and the efficient allocation of resources to properly address the health needs of a population. In this context, predictive and prescriptive analytics – known as advanced analytics — play a pivotal role in overcoming the ever-growing challenges of managing healthcare spending and cost containment. By identifying high-risk populations, tailoring care plans, offering predictive modeling, optimizing resource allocation, and enabling continuous improvement, it empowers healthcare stakeholders and organizations to make data-driven decisions that result in better patient outcomes and a healthier community.
Using Advanced Analytics with Risk Modeling
Risk modeling is a crucial component of public health initiatives, helping policymakers and healthcare professionals better understand and address the health challenges facing communities. Traditionally, these models have relied on descriptive data and statistical analysis to identify health risks and historical trends. However, the integration of predictive and prescriptive insights into these models can significantly improve their effectiveness in mitigating health risks, particularly for the most vulnerable.
Advanced analytics leverages historical and real-time data to identify individuals or communities at high risk for specific health conditions or diseases. By analyzing various data sources, including claims history, demographics, and social determinants of health, advanced analytics can pinpoint actual high-cost claimants and emerging-risk individuals who are most likely to develop certain health issues. This enables healthcare stakeholders to implement recommended targeted interventions, such as early screenings and preventive measures, for these high-risk populations.
This advanced form of risk modeling will be especially important as the burden of chronic disease and an increasingly aging population intensifies over the next decade and beyond. By 2030, one out of every five Americans will be 65 or older. Additionally, an increasing number of people are living with one or more chronic conditions. These converging forces will put additional pressure on an already strained healthcare system and exacerbate continuously rising costs. However, the industry can begin to shift away from reactionary methods and towards a proactive approach with prescriptive insights.
These benefits can be broadly applied across patient populations and areas of care where patients are most vulnerable to adverse outcomes, such as obesity or heart disease, to improve the understanding of care needs and prevent expensive emergent care. With advanced analytics, healthcare stakeholders obtain real-time feedback and performance metrics. By constantly analyzing the effectiveness of interventions and care plans, it enables healthcare stakeholders to make ongoing improvements to population health management strategies. This dynamic process allows for the continuous enhancement of care and the adaptation to changing healthcare needs.
Advanced Analytics in Value-Based Care
A key aspect of value-based care is tailoring care plans to individual needs. Predictive and prescriptive analytics can create personalized care plans for individuals based on their health history, preferences, and risk factors. This individualized approach to care improves patient engagement and adherence to treatment regimens. For instance, it can recommend appropriate treatment options, medications, and lifestyle modifications tailored to a patient’s specific needs. In consumer health, this tailored approach results in better health outcomes and reduced healthcare costs, as it minimizes trial-and-error treatment and hospital readmissions.
To understand the value of applying advanced analytics in this context, consider how employers traditionally approach benefit planning. Benefit plan design is typically centered on the expertise of decision-makers. Given the complexity of these products and possible combinations, plan designers resort to last year’s choices to inform the next designs iteratively. Employers also often offer additional wellness and preventive benefits to their plans, but the extent of these benefits can be cut short or limited due to budgetary considerations. Prescriptive analytics can provide recommendations that enable employers to optimally tailor the benefits portfolio to a specific subgroup of employees who may have different needs, rather than relying on a one-size-fits-all approach to provide the best health outcomes.
For example, members with musculoskeletal problems will be distinguished from those who require dietary support, and prescriptive methods would help employers best address those needs while maintaining budgets. Moreover, prescriptive analytics leverage past performance data to recommend the set of providers who would optimally improve health outcomes.
Healthcare organizations and members should look towards data science companies and payors who can combine an Area Under the Curve (AUC) methodology with large amounts of claims data to utilize historical data to accurately make predictions and provide patients and providers with actionable insights, mitigating risks and improving outcomes overall.
Advanced analytics can revolutionize the consumer health experience. When deployed more broadly, this technology will be instrumental in moving the needle toward proactively minimizing disparities and optimizing outcomes for all communities.
About Sean Crandell
Sean Crandell is SVP of Healthcare Economics at MultiPlan, overseeing the company’s Healthcare Economics team and is responsible for strategic analytic advisory regarding the delivery and design of healthcare services to our clients, provider partners, and MultiPlan stakeholders.