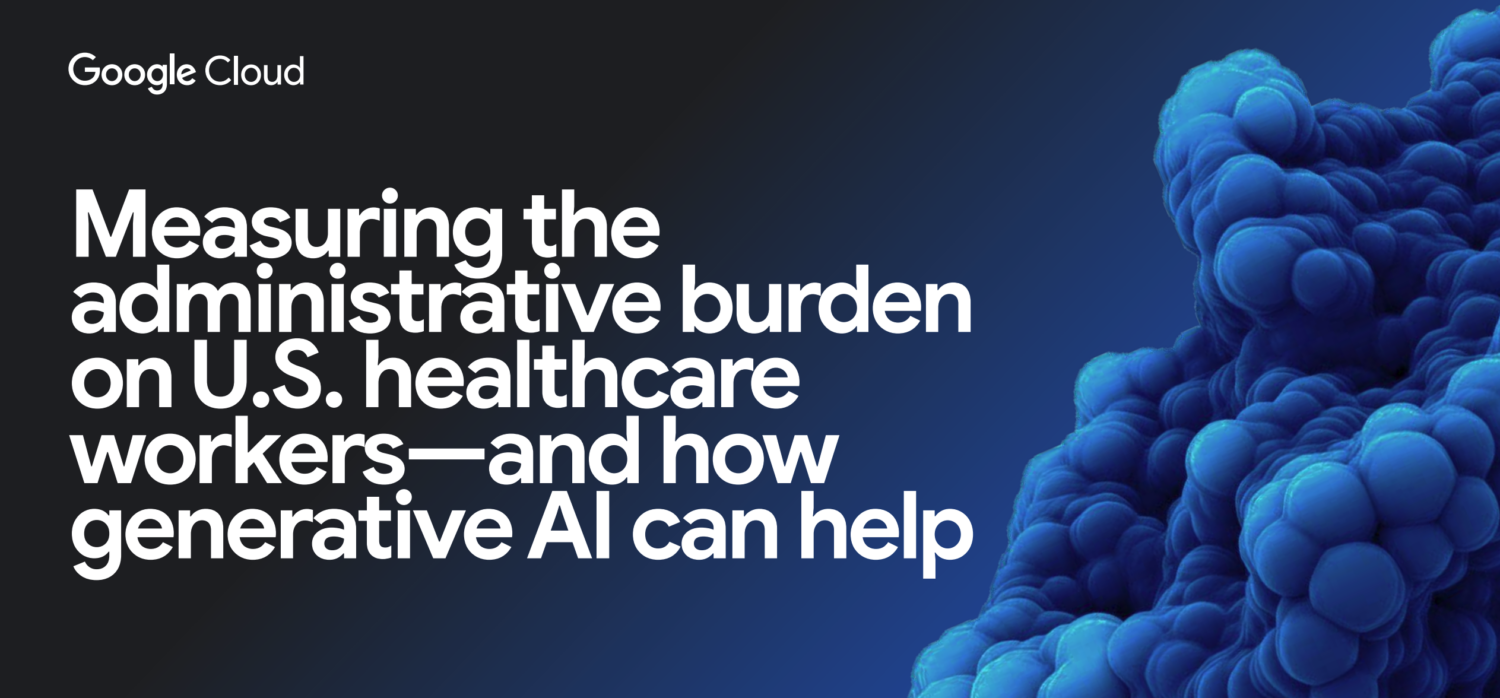
What You Should Know:
– A new research report from Google Cloud sheds light on the significant administrative burden faced by healthcare professionals and the potential for generative AI (gen AI) to alleviate these challenges.
– The report, “Measuring the administrative burden on U.S. healthcare workers—and how generative AI can help,” reveals that clinicians spend a substantial amount of time on administrative tasks, contributing to burnout, staff shortages, and reduced time with patients.
The Future of AI in Healthcare
The report suggests that generative AI has the potential to transform healthcare administration, reduce clinician burden, and improve patient care. However, it emphasizes the importance of responsible AI implementation with safeguards in place to protect patient data and ensure accuracy.
Key findings of the report include:
- Administrative Overload: Clinicians spend nearly 28 hours per week on administrative duties, while medical office staff and claims staff spend even more, averaging 34 and 36 hours per week, respectively.
- Burnout and Shortages: The majority of healthcare providers and payors agree that administrative work contributes to burnout and staffing shortages.
- Reduced Patient Care: 80% of providers report that administrative tasks take away from time spent with patients, impacting the quality of care.
- Increased Error Risk: Two-thirds of providers and 89% of payors express concern about human error in administrative tasks.
- Openness to AI: The vast majority of healthcare providers (91%) and payors (97%) are positive about using gen AI for administrative tasks.
Generative AI: A Potential Solution
The report highlights several ways generative AI can help streamline administrative tasks and improve efficiency in healthcare:
- Search and Summarization: Gen AI can intelligently surface relevant patient information from various sources and create concise summaries of clinical notes.
- Clinical Documentation: Gen AI can automate the creation of various clinical documents, such as discharge summaries and referral letters, freeing up clinicians’ time.
- Prior Authorization and Claims Processing: Gen AI can act as an intelligent assistant, pre-populating forms, analyzing requests, and suggesting relevant clinical guidelines.
- Radiology Workflow Optimization: AI can assist in analyzing medical images, helping radiologists make faster and more accurate diagnoses.
Real-World Examples
The report cites examples of healthcare organizations already leveraging generative AI:
- MEDITECH: Integrating AI-powered search and summarization capabilities into its Expanse EHR.
- HCA Healthcare: Developing a generative AI-powered nurse handoff tool to improve communication.
- Community Health Systems: Bringing gen AI to its tools to assist with administrative tasks.
- Waystar: Leveraging gen AI in its healthcare payments software platform to maximize reimbursements and prevent denials.
- Bayer: Developing an AI Innovation Platform to support the creation of AI-enabled apps for radiologists.
“Healthcare workers have historically faced significant administrative burdens, and this has intensified in recent years due to increased regulatory requirements, complex billing processes, and associated EHR documentation requirements,” said Aashima Gupta, global director of Healthcare Strategy & Solutions, Google Cloud. “But generative AI offers a powerful solution. By automating tasks and streamlining workflows, it supports healthcare experts, ultimately improving medical systems and helping doctors and nurses provide better care.”
Survey/Background Methodology
This survey was conducted online within the United States by The Harris Poll on behalf of Google from Aug. 26 – Sept. 9, 2024, among 821 healthcare providers, 209 payors, and 2,079 consumers aged 18 and older in the U.S. The sampling precision of Harris online polls is measured by using a Bayesian credible interval. For this study, the data for the healthcare sample is accurate to within + /- 3.4 percentage points, the data for the payor sample is accurate to within +/- 6.7 percentage points, and the data for the consumer sample is accurate within +/- 2.7 percentage points using a 95% confidence level.