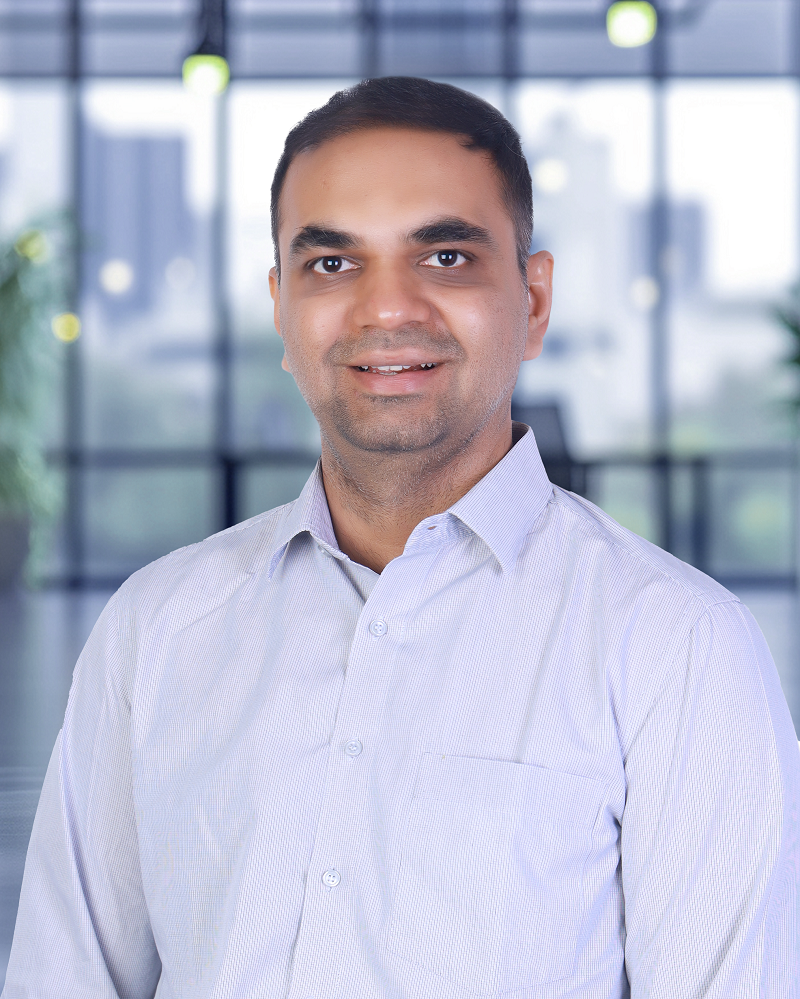
Administrative tasks are essential for ensuring high-quality patient care in health care, and the rehabilitation sector is no different. The sheer volume of patients can lead to an overwhelming burden of manual recordkeeping. Any inefficiency not only undermines the quality of care by causing delays for patients but also leads to therapist burnout.
It may be surprising to learn that, according to a U.S. clinician poll, 92% of clinicians agree that administrative stress is a major cause of burnout among health care workers. For 36% of practitioners, over half of their time is spent on administrative tasks. To address this stress, eliminate inefficiencies, or achieve other desirable outcomes, AI can be beneficial.
AI has become a potent instrument for simplifying administrative processes in the health care industry, providing innovative ways to automate jobs, enhance workflows, and boost productivity. After all, algorithmic rigor has the potential to unleash new efficiency wherever rote and repetitive administrative processes are present.
Before we shift all our attention to AI and laud its benefits, it’s essential to understand the underlying issues in rehabilitation management. Front office staff and other health care professionals are increasingly burdened with additional documentation, responding to patient correspondence, and coordinating with insurance companies. This growing administrative demand consumes time and attention, leaving less time for clinical patient care.
Let’s dig a little deeper.
The Data Management Struggles of Rehabilitation Administration
Rehabilitation administrators receive an overwhelming amount of data from various sources but struggle to extract valuable insights due to significant roadblocks:
- Paperwork Persists: While electronic health records (EHRs) exist, a significant amount of data still remains trapped on paper. Therapist notes, assessments, and intake forms create an inaccessible data graveyard, hindering analysis.
- Siloed Information Systems: Patient appointment histories and progress notes are often stored in separate systems, creating a fragmented data view. This makes it challenging to see the bigger picture and make informed decisions about patient care.
In addition, current methods for predicting patient needs are inadequate. Simple statistical models fail to capture the complexities of rehabilitation caseloads. They often overlook:
- Readmission Risk: The inability to anticipate patients likely to be readmitted hampers proactive staffing adjustments.
- Patient Acuity Levels: Inaccurate staff allocation results from a poor understanding of the severity of patients’ conditions.
- Therapist Skill Sets: Matching the right therapist with the right patient skill needs is crucial for optimal care, but current systems fail to identify potential skill gaps and tailor staffing accordingly.
Furthermore, reliance on manual audits for compliance creates a significant burden. These audits involve laborious, time-consuming, line-by-line reviews of patient records and documentation. This inherently manual process has several drawbacks:
- Inefficiency: Time spent on manual audits diverts administrators from essential tasks like staff development and patient care initiatives.
- Human Error: Missed details buried in voluminous patient records are a risk with manual reviews, potentially leading to undetected compliance issues with severe consequences.
- Workflow Disruption: Manual audits disrupt the entire facility’s workflow as staff are pulled away from their regular duties to assist with the process.
AI’s Transformative Impact
Adam Spielman of Citi Global Insights examines in “Smart Thinking on AI in Healthcare” how AI-driven automation could decrease administrative expenses by 25% to 30% while benefiting staff, enhancing patient experiences, and saving time and money.
Here is how:
Predictive Analytics and Patient Flow Optimization
Traditionally, predicting patient demands in rehabilitation has been a challenge. Factors like the number of patients, therapist workloads, and patient understanding are complex and interrelated. This is precisely where AI excels. AI-powered systems can analyze massive datasets containing this historical information, enabling them to forecast future patient demands accurately in:
- Dynamic Appointment Scheduling: AI can anticipate upcoming patient volumes and adjust therapist schedules accordingly. This ensures appointments are readily available for patients while preventing therapist overload during peak periods.
- Proactive Resource Allocation: By predicting patient readmission risk, AI empowers administrators to anticipate staffing needs. Resources can be proactively allocated to ensure smooth care transitions for patients returning to the facility.
- Reduced Wait Times: AI-powered scheduling minimizes patient wait times, leading to a more positive and efficient patient experience.
Example: Seasonal falls have typically caused a rehabilitative facility’s patient load to spike in the winter. An AI system can analyze this pattern, which can then forecast an increase in lower limb fracture patients. This enables managers to proactively modify therapists’ schedules, guaranteeing enough personnel to manage the projected caseload and reduce the time it takes for appointments.
Data-Driven Decision-Making
Despite being inundated with data, rehabilitation managers sometimes lack the resources to glean insightful information, providing a potent remedy by sifting through enormous datasets to spot patterns, forecast results, and guide fact-based administrative choices. How to do it is as follows:
- Identifying Patients at Risk: AI can analyze patient data to identify individuals at higher risk for developing complications based on medical history and current presenting conditions. This allows for proactive interventions and targeted care plans, potentially improving patient outcomes.
- Fraud Detection and Prevention: AI can analyze billing data to identify patterns suggestive of fraudulent claims. This empowers administrators to implement preventative measures and ensure proper reimbursement for services rendered.
Example: An AI system examines patient data to spot trends in the rise of opioid prescriptions for particular diseases. By highlighting possible abuse and encouraging administrators to investigate further, this data may help stop prescription medication abuse.
AI-Powered Reimbursement Optimization
The healthcare sector is known for its complicated reimbursement procedures, which include fines for mistakes and lost chances. AI can simplify the coding and billing process, completely changing it.
- Automated Coding: AI can analyze patient records and automatically generate accurate and complete medical codes, eliminating the risk of human error and ensuring proper reimbursement.
- Missed Billing Opportunity Detection: AI can identify services rendered that haven’t been coded or billed for, maximizing revenue capture for the facility.
Example: An AI system detects a pattern in which therapists routinely undercode a certain therapy modality because the billing criteria are unclear. With this data, managers can guarantee that all treatments are appropriately recorded for billing purposes and offer therapist-focused instruction.
The difficulties that rehabilitation administration faces are significant but addressable. In fact, they present a strong opportunity for transformative progress. Using AI, employers and workers will profit from increased cost savings, improved user experience, and patient-centered treatment. Moreover, administrative procedures may be continually improved and adjusted using machine learning models. This means that AI systems become better at handling complex tasks as more data is analyzed, ultimately reducing the need for human intervention to the bare minimum.
However, it’s important to remember that AI is here to augment, not replace, the human workforce in administration. AI’s true strength lies in its ability to automate repetitive tasks and meticulously analyze data, but at the end of all that, human expertise is essential. In all cases, AI will function as a potential ally to empower rehab therapists, and this empowered workforce will ultimately drive superior patient outcomes, solidifying rehabilitation centers as bastions of high-quality care.
About Brijraj (Vaghani) Bhuptani
Brijraj (Vaghani) Bhuptani is co-founder and chief executive officer of SPRY Therapeutics, Inc., inventor of rehab therapy’s first fully integrated, AI-powered EMR. As CEO, Brij drives company and product strategy as he leads the organization in the commercialization of rehab therapy’s only AI-first software platform. Before SPRY, Brij co-founded and served as chief executive officer of Birds Eye Systems, the creator of major mass transit platform Ridlr. This enterprise was acquired by Ola, one of the world’s largest ride-hailing companies, where Brij then served as chief technology officer. Prior to Birds Eye Systems, Brij applied his engineering background to solving some of the most pressing technology concerns facing large media and wireless firms, including Qualcomm and Sears India.