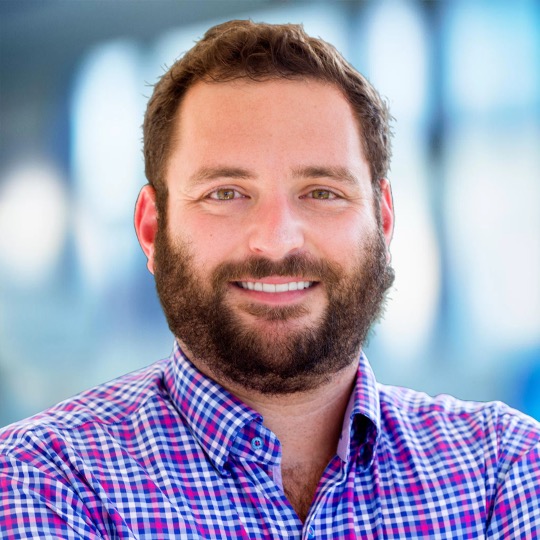
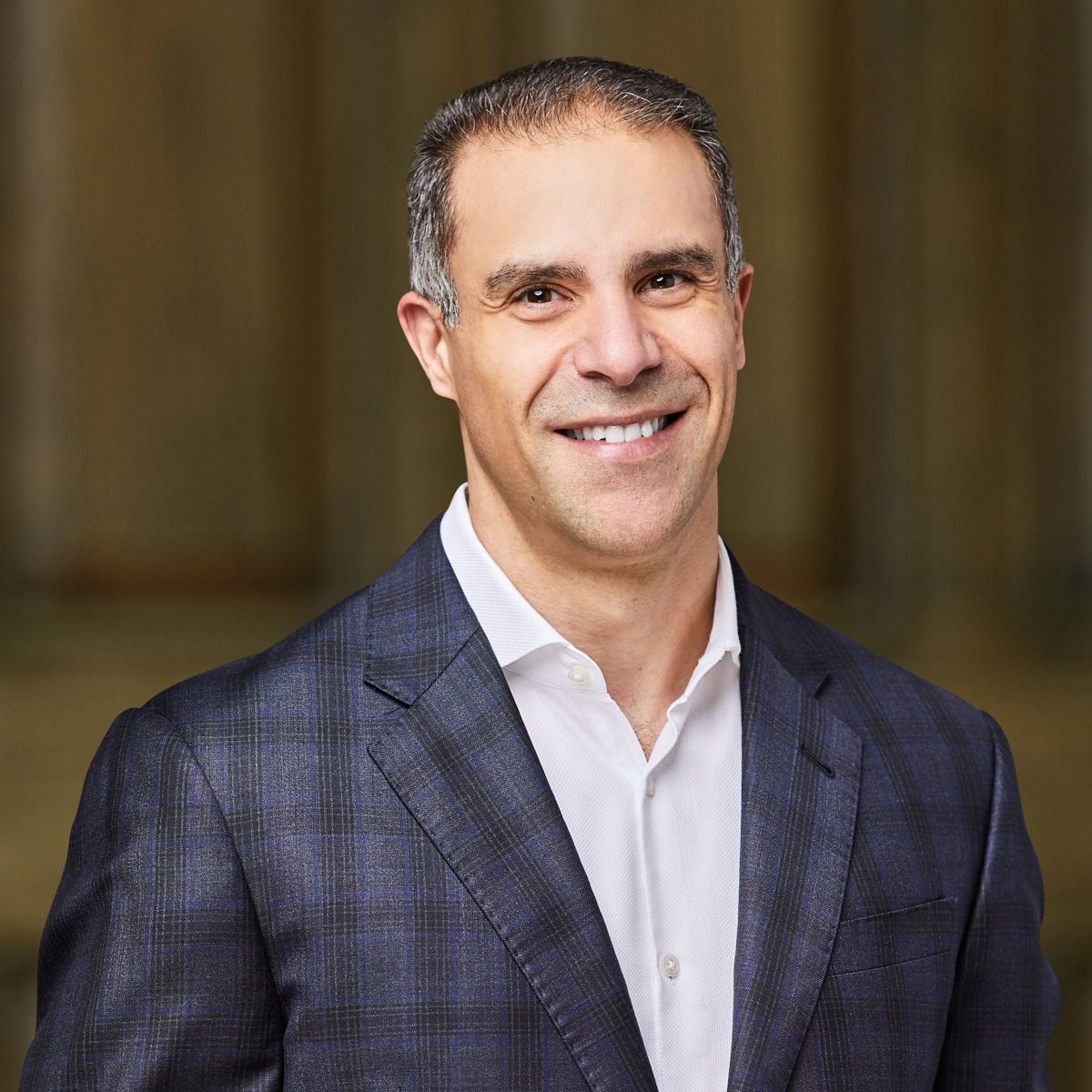
It’s human nature that when we are told to do something, we want to know the reason why.
That’s especially true when the direction comes from a machine, and it concerns something as important as healthcare. However, some artificial intelligence (AI) systems being used in healthcare do not offer that option; instead, they issue generic prompts with limited transparency into the reasoning behind it.
In contrast to these large language-based models of AI, other systems emphasize explainability and are guided by clinical expertise, ensuring safer and more reliable decision-making.
As AI and large language models (LLMs) become more integrated into healthcare, it is essential to develop frameworks that prioritize patient safety, clinical expertise, and evidence-based practice. A reasoning-based approach to AI offers a novel pathway, leveraging the power of AI while ensuring its recommendations remain grounded in medical knowledge.
3 Core Principles of Responsible AI Use
The reasoning-based approach to AI-enabled care management has three components, all of which enhance care transitions and improve patient outcomes:
- Physician-developed AI logic (AI inputs) – Responsible AI systems use physician-guided reasoning to structure outputs, rather than relying solely on impenetrable black-box algorithms. The foundation of this approach is a comprehensive set of risk factors and interventions, defined and validated by experienced physicians. These risk factors are rooted in specific diagnostic criteria and medical metadata, aligning closely with the latest clinical guidelines and peer-reviewed literature. By anchoring AI outputs in a clinician-driven knowledge base, the system ensures that its recommendations reflect current medical standards and are tailored to the complexities of patient care.
- Structured intervention framework (AI outputs) — AI-generated interventions are broken down into clear, specific steps. Each action includes a priority level, a clear description, interaction types, the designated healthcare professionals responsible for the action, and recommended tools. This structured framework ensures that recommendations are practical, easily implementable, and aligned with the workflows of care teams.
- Multidisciplinary Integration: Interventions often require action from a range of healthcare professionals, including nurses, physician assistants, care managers, and specialists such as cardiologists or pulmonologists. This multidisciplinary approach acknowledges the complexity of patient care and ensures that AI recommendations can be integrated seamlessly into care team structures, supporting coordinated and comprehensive treatment plans.
Advantages of a Reasoning-Based AI Approach
The reasoning-based approach offers several distinct advantages over traditional LLM prompting:
- Controlled inputs: This AI operates within a clinically validated framework of risk factors and interventions, which minimizes the risk of irrelevant or inaccurate outputs. This controlled environment ensures that prompts remain focused on patient-specific conditions and needs.
- Transparency to clinical logic: One of the major challenges with LLMs is that their black box nature makes it difficult to see the reasoning behind recommendations. In contrast, this reasoning-based approach provides full transparency, with each AI-generated recommendation traceable to specific evidence-based clinical guidelines. This makes the decision-making process clear, auditable, and easier for clinicians to trust and implement. Without clear explainability, it is unlikely that clinicians will adopt any new tool, especially one powered by AI.
- Clinician oversight: AI streamlines and augments the identification of risks and recommendations, but never replaces clinical judgment. This system relies on human oversight for the definition, review and updating of risk factors and interventions, ensuring that medical expertise remains central to patient care.
- Standardized outputs: The structured intervention framework ensures that AI-generated outputs are consistent, actionable, and ready for immediate integration into clinical workflows. By standardizing the outputs, the system helps maintain quality and alignment across different care teams and settings.
- Reduced risk: By basing the AI’s analysis on predefined, clinically validated content, this approach significantly reduces the risk of AI-generated errors, “hallucinations,” or incorrect recommendations. That’s a critical improvement over LLMs that generate outputs based on less structured inputs (prompts and clinical data), which can introduce variability and potential inaccuracies.
Applications in Care Transition
The three principles of responsible AI use are particularly applicable in care transitions, where managing patient handoffs between different care settings is critical. AI-driven platforms can harness this reasoning-based approach to improve coordination, activate appropriate interventions, and prevent adverse events such as readmissions or emergency department visits.
The importance of deploying responsible AI frameworks clinicians trust cannot be overemphasized. Responsible, transparent and safe AI can be applied in real-world care management applications. By combining AI with rigorous clinical oversight, it’s possible to enhance patient outcomes while also maintaining high standards of safety, explainability, and accountability.
As AI continues to shape the future of healthcare, adopting a reasoning-based approach that emphasizes clinical expertise, evidence-based practice, and patient safety will be essential for realizing the potential benefits of this technology while mitigating risks. By anchoring AI outputs in clinician-driven knowledge and maintaining transparency in its decision-making, this approach provides a more reliable, explainable, and ultimately safer framework for using AI in complex clinical environments like care transitions.
About Matt A. Murphy
Matt A. Murphy is the CEO and Co-Founder of Cascala Health, a digital health startup using Clinically Responsible AI to transform care transitions, streamlining care team engagement and information flow so patients don’t disengage or get lost between handoffs. A dedicated healthcare innovator, Matt has a strong track record of building and scaling digital health companies. He previously held leadership roles at Cohere Health, Circulation Health, and ModivCare (NASDAQ: MODV), where he drove significant growth and transformative clinical solutions. Also an advisor to early-stage digital health startups, Matt started his career at Boston Children’s Hospital, where he launched its first digital health accelerator.
About Joe JasserJoseph Jasser, MD, MBA is a seasoned C-Suite healthcare executive with over 20 years of experience within the healthcare industry and a proven background in multiple roles, including founder, CEO, COO, and CMO. He currently serves on the board of Cascala Health and has held previous executive roles at Cleerly, Elara Caring, Humana, Signify Health, Dignity Health, and Cigna, where he built data infrastructures, launched innovative care models, and managed high-performing clinical teams.