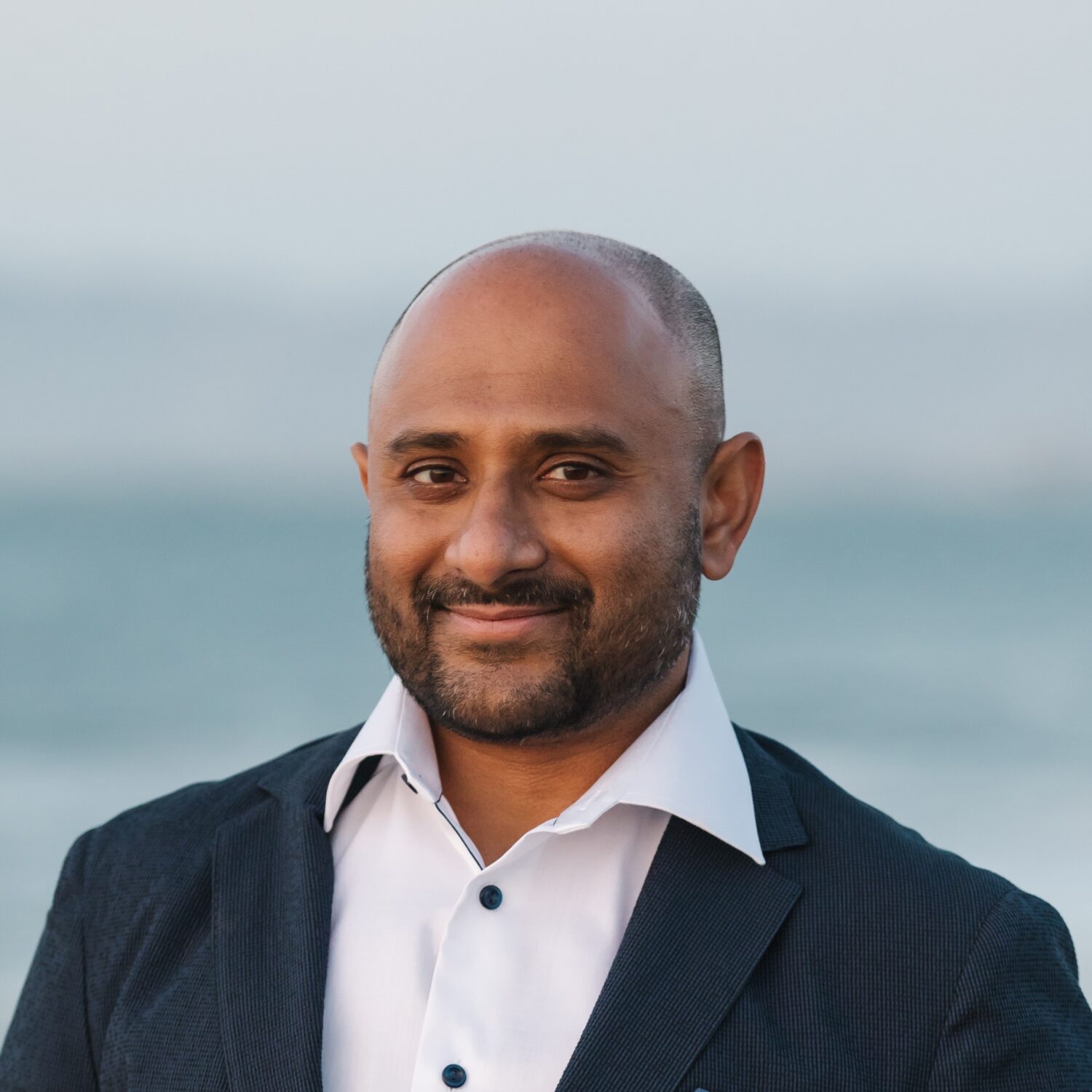
The integration of AI into healthcare workflows presents a tremendous opportunity for transformation –– and for new levels of productivity in a time when administrative functions surrounding claims adjudication, provider reimbursement, and related functions are complicating operations and affecting efficiency. AI Co-Pilots offer an innovative approach to addressing these issues by driving efficiency, accuracy, and value in payment integrity.
The payment Integrity sector, crucial for ensuring accurate claim settlements and proper reimbursements, is valued at $9 billion by McKinsey & Co., and has been growing at a 7% annual rate, driven by increased healthcare spending and complex billing procedures.1 Today, payment integrity involves both prepayment and post-payment capabilities. On the prepay side, it’s important to keep on top of ever-changing policies –– which is vital to the claim and the adjudication process. There are policies from CMS, HHS, states, and payers themselves come up with policies. Then there’s the claims editing process.
How do you make a decision when a claim comes in?
Ultimately, it’s all influenced by understanding the legalese and contractual language and translating those into actionable rules., It’s best practice to review both current as well as updated rules and policies—making them actionable in payment integrity activities. However, there are literally hundreds of thousands of documents that change each year–– making it nearly impossible for humans to keep up.
This is a prime application for large language models (LLMs), which have unlocked our ability to understand language more fundamentally. These models, which include GPT, Megatron, and others, serve as foundational tools, enabling the creation of sophisticated AI applications tailored to specific needs in healthcare—including payment integrity and claim processing. Applying LLMs to better understand all of these constantly changing documents, then reason with the content, and build experiences, is one example of an AI Co-Pilot. A Co-Pilot is just what the name implies – an agent that allows us to build solutions to help users in the payment integrity space, without changing their workflows.
What Role Do AI Co-Pilots Play in Payment Integrity?
Simply stated, AI Co-Pilots act as intelligent assistants embedded within current workflows and systems, designed to enhance human capabilities through greater accuracy and efficiency. LLMs allow Co-Pilots to analyze policies, guidelines, patient data, and other unstructured information to extract insights and “reason” with the data. Their real-time insights can then assist clinicians and staff in navigating complex decisions related to claim approvals and treatment plans.
Co-Pilots have the capacity to enhance productivity and compliance without disrupting existing workflows. By providing personalized, context-aware guidance, Co-Pilots help streamline decision-making processes and simplify the management of evolving regulations. For instance, they can monitor updates to payer policies and alert staff to the implications for claim assessment, reducing the burden of staying up to date on complex regulations.
Driving Operational Efficiency
Studies have demonstrated that AI Co-Pilots can significantly improve efficiency in eye-opening ways. In payment integrity, they automate the labor-intensive task of reviewing numerous documents to identify policy changes relevant to claim approval. By rapidly surfacing pertinent information, Co-Pilots accelerate informed decision-making and enable faster adjudication.
One example is reducing policy review time from months to weeks through the assistance of a Co-Pilot, resulting in lightning-fast quantifiable time and cost savings. When it comes to clinical policies that a typical state Medicaid publishes, on average, there are more than 5,000 documents that a clinician must first sample and then evaluate for relevancy. Each relevant section must be read before deciding if it applies to very specific rules and edits. Everything then must be codified. This can take months, if not years! 50,000 documents are impossible for humans to review, and if you multiply that by 50 states, there are millions of documents that are highly relevant to healthcare costs, care, and stakeholder interaction.
Each year, at least 20% to 25% of these documents change –– and there’s no pattern or way to predict which ones it will be. With LLMs, you can automate the downloading of these documents, and then understand which ones are relevant by having many smaller LLM models that indicate which ones are clinically relevant. LLMs can then extract the relevant section and deliver only the contextually pertinent content to clinical teams to apply their expertise. Humans remain the experts, but the LLM makes it easier for them to know where to focus their attention.
Another type of a Co-Pilot uses experiential data –– say, from customer service representatives, providers, and members –– to improve member experiences. For example, if a member inquires about a claim that was denied, this data could help provide a non-technical, plain-language explanation for the action. Unlike a sterile chatbot, this approach helps simplify the business of care, boost member satisfaction and reduce provider abrasion by helping the member or provider understand the reasons for the action, find better care, and gain transparency into the associated rules.
The substantial productivity gains achievable through Co-Pilots can lead to significant cost savings when implemented across large healthcare systems. By automating repetitive tasks, Co-Pilots free up resources that can be reallocated to higher-value work. As healthcare becomes more competitive, AI automation becomes increasingly essential for controlling expenses and optimizing resource allocation.
Integrating AI without Disruption
The Co-Pilot approach emphasizes enhancing workflows through unobtrusive integrations within familiar platforms such as MS Word or claims management software. By providing in-context help and guidance, users can benefit from AI assistance without needing to change established habits. This “human-in-the-loop” model maximizes trust and impact, ensuring smoother adoption of AI technologies in healthcare settings.
Overcoming Industry Challenges with AI
Co-Pilots address long-standing healthcare challenges by combining human expertise with data-driven intelligence. The utilization of natural language processing enables the parsing of complex policies at scale, while machine learning integrates disparate data sources to create actionable profiles. Additionally, lightweight workflow integrations simplify the adoption. Measures can be taken to address potential algorithm bias and accuracy issues, like building test datasets to evaluate model performance.
Pioneering Human-AI Collaboration
To fully realize the benefits of AI in healthcare and ensure improved access, affordability, and quality of care, health plans must pioneer human-AI collaboration models that prioritize payment integrity. Co-Pilots can be customized for different applications and use cases, using foundational and smaller specialized models tuned to different domains and data sets. The transformative power of AI Co-Pilots lies in their ability to elevate human capabilities. Conversational AI/”agents” could be used to automate and streamline processes further in the future.
AI Co-Pilots exemplify the transformative change possible by combining human expertise with data-driven intelligence. By automating repetitive tasks and seamlessly integrating into workflows, Co-Pilots accelerate innovation in healthcare. Continued exploration of human-AI collaboration models is crucial to realize improved access, affordability, and quality of care as we enter the era of AI in healthcare. With deliberate and ethical AI implementation, healthcare knowledge, productivity, and patient outcomes can be significantly enhanced.
About Akshay Sharma
Akshay Sharma, Chief AI Officer of Lyric, brings a rich tapestry of expertise and entrepreneurial spirit to the forefront of healthcare innovation. His leadership focused on driving Lyric’s AI advancements, enhancing product and technology within the Lyric platform. Sharma spearheads artificial intelligence initiatives, guiding research & development and the strategic direction of AI services and solutions. Previously, Akshay was an Entrepreneur In Residence at Elevance Health, EVP of AI at Sharecare, CTO at doc.ai, and Head of Product and Engineering at Human API.