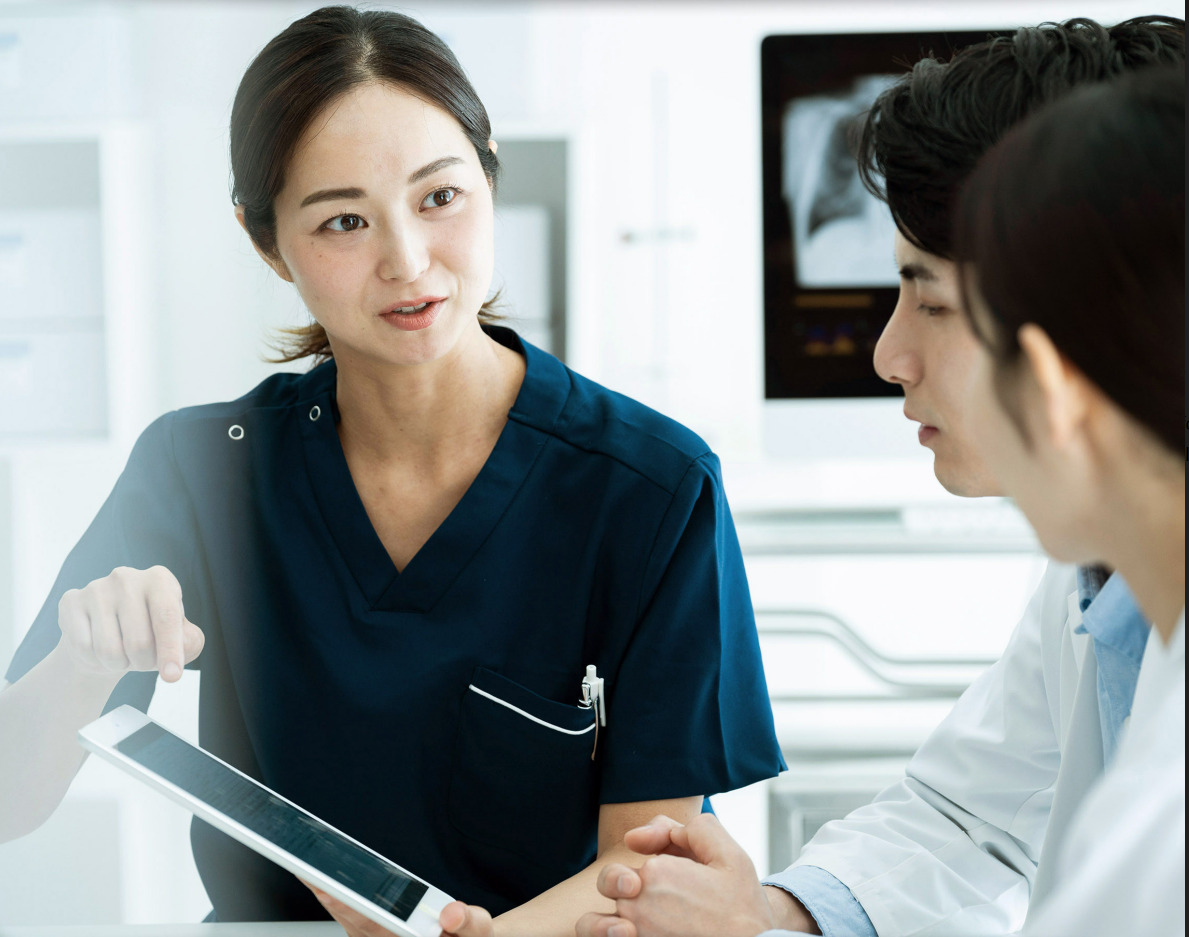
What You Should Know:
- Signal (Epic’s provider-efficiency tracking tool) is a familiar sight at many organizations using the Epic platform—these organizations harness Signal data to understand the diverse and complex ways end users interact with the EHR.
- They often use Signal data to pinpoint providers who may be struggling and could gain the most from EHR training interventions.
Examining Signal’s Implications for HER Satisfaction, Burnout and Turnover
Many Arch Collaborative members ask KLAS whether Signal data can predict a provider’s EHR satisfaction, degree of burnout, or likelihood to leave the organization. Current analysis of Collaborative data confirms past findings: while Signal data has many effective and valuable uses, it is not a meaningful or predictive measure to discern whether providers are dissatisfied with the EHR, experiencing burnout, or considering leaving the organization.
Key findings from the report are as follows –
- Signal Helps as a Conversation Starter—but Not as a Predictor of EHR Dissatisfaction, Burnout, or Turnover: Signal data is not a reliable predictor of individual provider EHR satisfaction. The discrepancy is due to differences in data collection and what they measure. Epic Signal data reflects system usage, making disengaged users appear similar to efficient ones. In contrast, Arch Collaborative data assesses overall EHR perception over time. However, Signal data can still be useful as a starting point for improving the EHR experience. High-performing Arch Collaborative members use Signal data to initiate conversations and interact with providers effectively.
- With Small Statistical Correlation, a Few Signal Metrics May Be Helpful Markersfor EHR Experience: In KLAS’s predictive algorithm, a few Signal variables have a modest yet statistically significant impact on the EHR experience. These variables include the time providers spend in the EHR per day during scheduled hours, which relates to overall EHR satisfaction (r2=0.03), and the time spent on In Basket tasks per day, which is linked to burnout (r2=0.02). Furthermore, specific metrics within the NEES were analyzed, such as ease of learning, efficiency, and specialty-specific functionality. For ease of learning and efficiency, the most critical Signal metric is the time spent in the EHR per day during scheduled hours. When it comes to satisfaction with specialty-specific functionality, the key Signal metric associated with success is the time spent on orders per day.