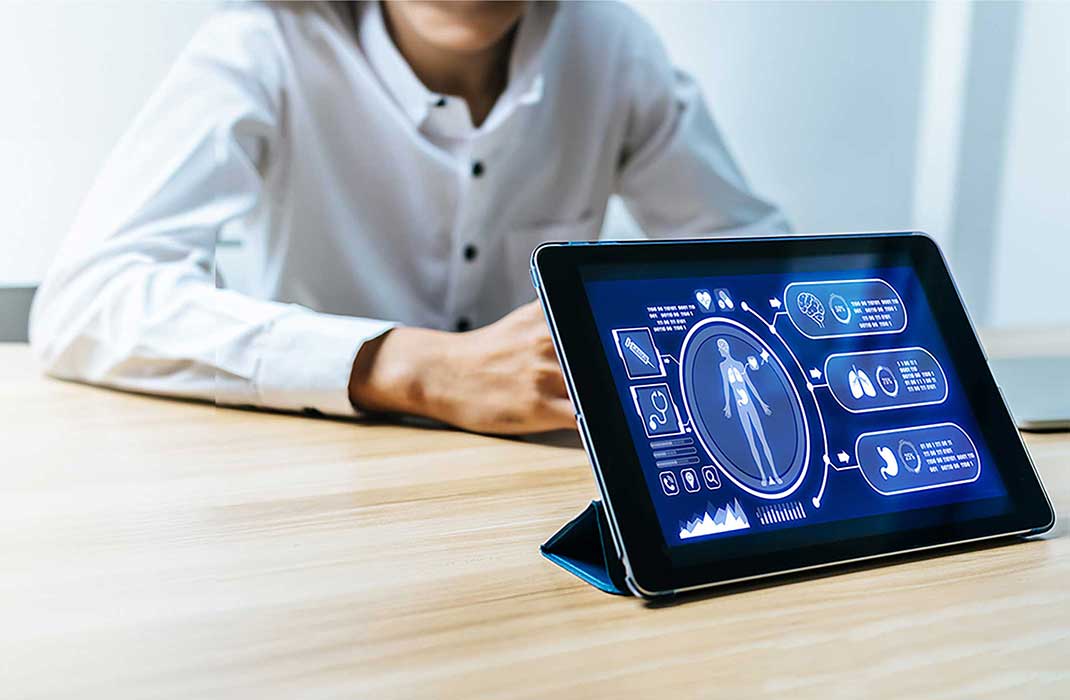
What You Should Know:
– Researchers at Mass General Brigham have developed two powerful AI models, UNI and CONCH, that represent a significant leap forward in computational pathology (CPath).
– Published today in Nature Medicine, the two new foundation models, trained on massive datasets, hold immense potential for improving diagnoses, predicting patient outcomes, and even identifying rare diseases.
What are Foundation Models?
Foundation models are advanced AI systems trained on vast amounts of data. This training allows them to learn complex patterns and relationships within the data. In CPath, foundation models are trained on pathology images, often accompanied by corresponding medical text descriptions. This empowers them to analyze tissue samples, identify abnormalities, and glean insights from associated reports.
Understanding UNI and CONCH Foundational Models
- UNI: This model focuses on understanding pathology images, excelling at tasks like disease detection and whole slide image analysis. Trained on over 100 million tissue samples, UNI leverages transfer learning to apply its knowledge to various clinical scenarios. It outperformed existing models in 34 tasks, showcasing its adaptability and potential as a versatile CPath tool.
- CONCH: This unique model integrates image and text data, allowing pathologists to interact with it using medical terminology. This enables CONCH to excel at tasks like identifying rare diseases, segmenting tumors, and understanding complex whole slide images. In 14 clinical evaluations, CONCH surpassed standard models, demonstrating its effectiveness and versatility.
UNI and CONCH Foundational Model Benefits and Future Implications
These foundation models offer several advantages:
- Improved Diagnostic Accuracy: UNI and CONCH can potentially lead to more accurate diagnoses, aiding pathologists in complex cases.
- Enhanced Prognostic Insights: The models may provide valuable insights into disease progression and patient outcomes.
- Rare Disease Identification: CONCH’s ability to analyze text descriptions might prove crucial in identifying rare diseases with limited visual data.
Availability
The research team is making the code publicly available, encouraging further development and application in tackling real-world clinical problems. This marks a significant step towards a new era of AI-powered CPath, with the potential to revolutionize medical diagnosis and patient care.
“Foundation models represent a new paradigm in medical artificial intelligence,” said corresponding author Faisal Mahmood, PhD, of the Division of Computational Pathology in the Department of Pathology at Mass General Brigham. “These models are AI systems that can be adapted to many downstream, clinically relevant tasks. We hope that the proof-of-concept presented in these studies will set the stage for such self-supervised models to be trained on larger and more diverse datasets.”