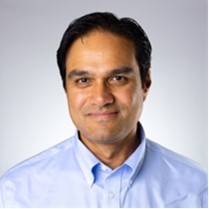
While most technology- and innovation-oriented industries have followed the general principles of Moore’s Law, which holds that computing power would double yearly, while costs were cut in half, the life sciences industry appears to be spending more money developing fewer drugs.
According to the latest data, the cost to take a drug candidate from the discovery stage, all the way to market launch, hit $2.3 billion in 2022—a $298 million rise from 2021. Meanwhile, there were just 37 novel drugs approved for the year, the fewest since 2016. While part of that is attributable to the unique nature of drug development, versus other industries, and the enormous stakes at play if researchers, manufacturers and regulators fail to hit the mark, there are some lessons on innovation and efficiency that could be learned from other industries.
A Model Ripe for Reinvention
Let’s look at clinical trials, for example. The randomized controlled trial has always been considered, among many, to be the gold standard of scientific research because it represents the very foundation of the scientific method applied in a real-world setting. However, just because the base methodology of the trial itself needs to be followed, doesn’t mean we cannot innovate the processes through which clinical sites are selected, patients are recruited and data is collected and analyzed.
Starting with clinical trial site selection, the age-old process of designing and planning a clinical trial—which, in most cases, has not evolved for the last two decades—involves a pharmaceutical sponsor working with a contract research organization (CRO) to identify several clinical sites from their clinician networks. The next step is recruiting patients through the clinicians and direct-to-patient advertising in regions where those sites are located. This approach ends up prioritizing the same handful of sites in which CROs have already established relationships and results in most studies that are being conducted in major research centers throughout large cities.
Although that has been the industry standard approach, it may not be the most accurate or efficient way to design a study. Additionally, it’s an inherently biased process that disproportionately favors large hospital and clinician groups in major metro areas. It’s also extremely difficult to design trials that will fully mirror real-world patient populations because they are such controlled environments with a limited sample size. For instance, when we look at the real-world application of faricimab for wet age-related macular degeneration (AMD), we see that the real-world population has many more patients starting on faricimab that previously received anti-VEGF treatment, than treatment-naive) patients. Additionally, in clinical trials, patients adhere to a very strict dosing interval that is often not strictly replicated in the real-world, thereby extending the dosing intervals.
Leveraging Real-World Evidence from the Start
Thanks to recent advances in real-world evidence (RWE) and artificial intelligence-powered analytics, it is possible to dramatically improve the precision of this process by using patient experiences, instead of legacy relationships, to guide site selection. It is possible, for example, to use carefully curated electronic health record (EHR) data to quickly identify practices with clinical expertise and patient populations matched to unique study protocols.
By reorienting the site selection and patient recruitment process around specific patient cohorts, sponsors and CROs can cut timelines and improve accuracy. Still, many are continuing to follow old processes and spinning their wheels after realizing their study populations are not fully aligned with the real-world patient pool.
For example, a phase two trial for wet age-related macular degeneration (wet AMD) was at risk of missing its patient recruitment goals with just five weeks remaining in the trial, but by utilizing patient identification services to support site selection and patient pre-qualification, the trial was able to meet the recruitment goal.
In these instances, the trials were already underway, and everything needed to be retooled and reprogrammed while in flight. While this was a success and a stage failure was avoided, it would have been a lot easier and more effective to start with the data-driven approach from the outset to de-risk and guide the process from the beginning.
AI-enabled analytics can unearth new clinical trial sites in extremely narrow therapeutic areas. Thyroid eye disease, for example, is an exceedingly rare condition for which several active clinical trials are running, making patient recruitment extremely challenging. By flipping the conventional script on site selection and using RWE to guide clinician identification and patient recruitment, it is possible to identify under-the-radar sites that would have never been found using conventional, key opinion leader (KOL)-driven methods.
Moving Science Forward with Integrity
Examples, like these, are occurring every day in the new drug development pipeline. There are antiquated approaches to KOL research, cumbersome and costly patient recruitment strategies, and myopic approaches to trial results that don’t take into account RWD from outside the trial. It is possible to innovate the process without compromising the integrity of the science behind it. It is essential in order to move science forward.
We are all living and working in a unique moment in history where the power of technology to transform legacy processes and create new opportunities, may have finally caught up with the ideas we could only dream about a few years ago. In addition, now there are experts with highly developed skill sets in RWE and analytics who’ve spent their entire academic and professional lives in a world where virtually unlimited data and boundless computing power have made it possible to answer previously unanswerable questions. We owe it to future generations to begin leveraging that technology, and those amazing minds, to find cures faster.
About Sujay Jadhav
Sujay Jadhav is the Chief Executive Officer at Verana Health where he is helping to accelerate the company’s growth and sustainability by advancing clinical trial capabilities, data-as-a-service offerings, medical society partnerships, and data enrichment.