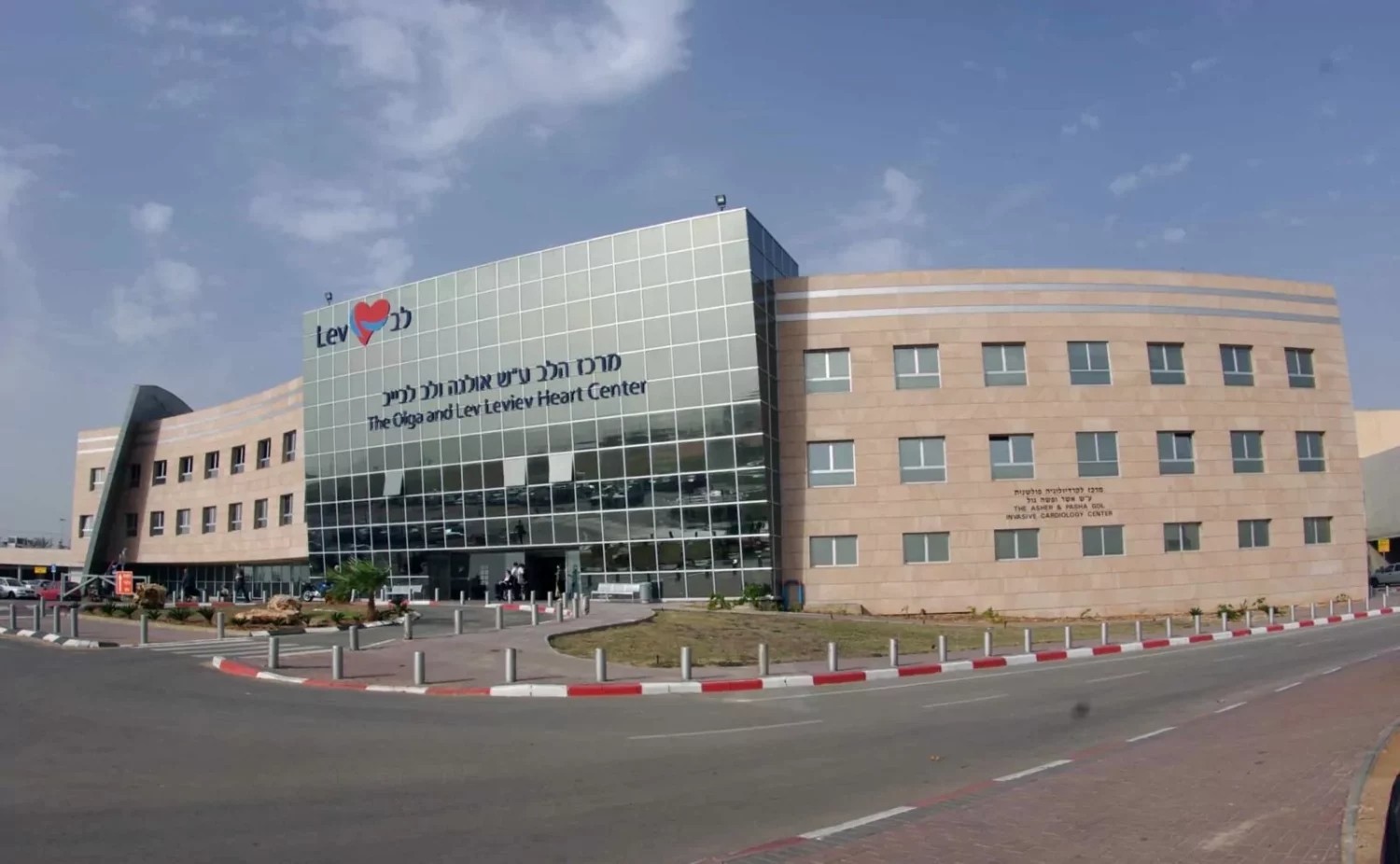
What You Should Know:
– Sheba Medical Center, a globally recognized healthcare leader, announced the publication of a groundbreaking study demonstrating the effectiveness of an AI algorithm in predicting pulmonary embolism (PE) risk upon hospital admission.
– The research, published in the Journal of Medical Internet Research, involved analyzing medical records of over 46,000 patients and identifying a machine learning model capable of accurately predicting PE risk.
Understanding Pulmonary Embolism
Pulmonary embolism, a potentially fatal condition caused by a blood clot blocking a lung artery, remains a significant challenge in healthcare. Traditional diagnosis methods rely on CT scans and clinical judgment, which can lead to delays in treatment. Sheba’s AI algorithm offers the potential to improve early detection and intervention significantly.
Led by Prof. Gad Segal, Head of the Sheba Education Authority, in collaboration with researchers from Ben-Gurion University, the study successfully trained a random forest algorithm to identify patients at high risk of PE based on their medical history. This breakthrough has the potential to revolutionize PE diagnosis and treatment, enabling clinicians to initiate timely interventions and improve patient outcomes.
AI Model Integration
Sheba Medical Center plans to integrate this AI model into its clinical workflow alongside other diagnostic tools to create a comprehensive risk assessment platform. By combining advanced analytics with clinical expertise, the hospital aims to further enhance patient care and reduce the burden of PE.
“Early and timely diagnosis of pulmonary embolism is challenging, yet crucial, due to the condition’s high rate of mortality and morbidity,” said Prof. Gad Segal. “This study highlights the enormous potential of machine learning tools to support innovation in diagnostics. Even though the model only used data available from patients on arrival to the ER, it was still able to predict with high accuracy the likelihood a patient developing PE, a crucial advancement for patient care and outcomes.”