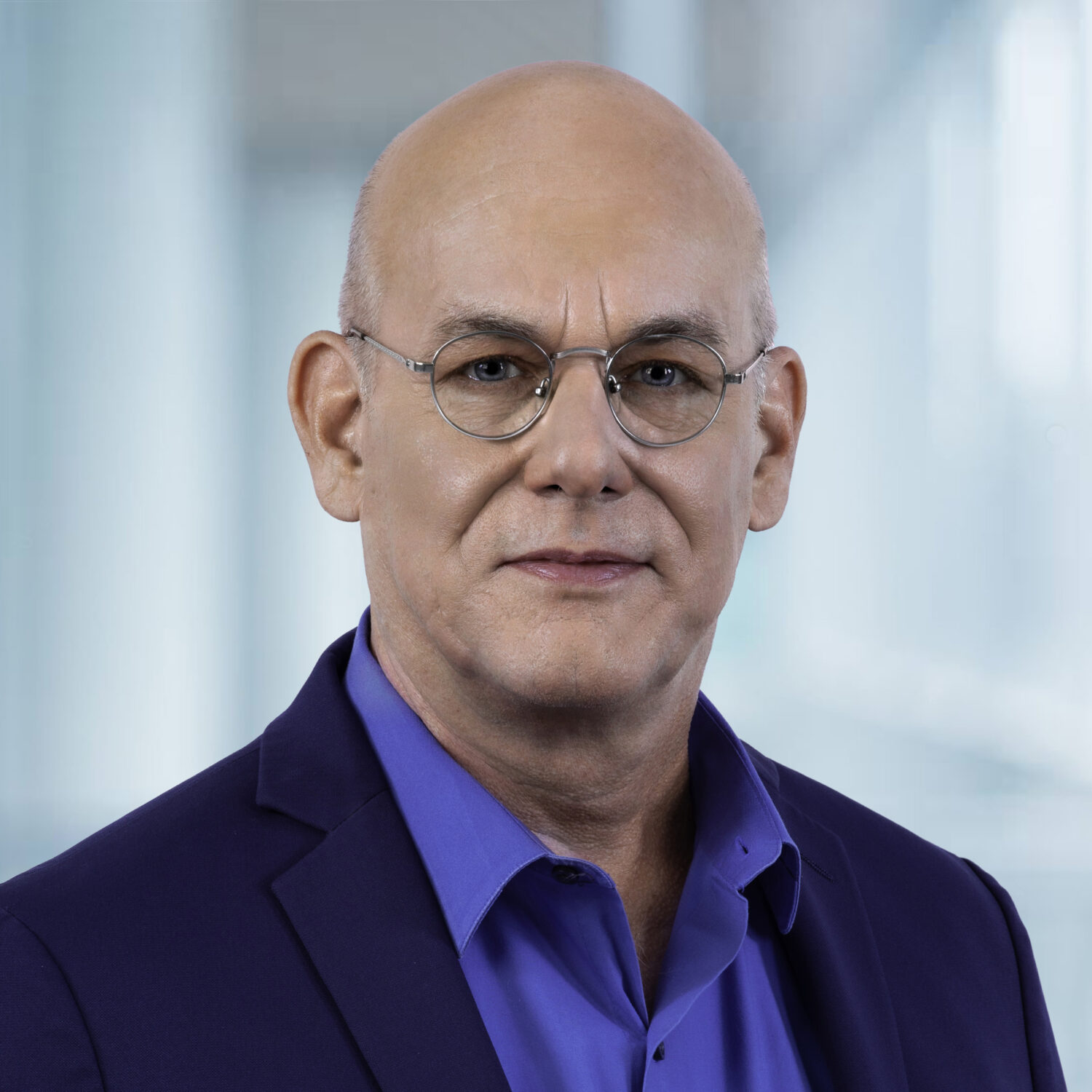
Healthcare providers face staffing shortages, increasing regulatory requirements, and pressure to reduce costs while delivering effective care. Compounding provider problems are payor policy, behavior changes and ongoing fee schedule cuts that compress revenue, as well as mounting denials and additional documentation requests from payors.
Coding, claim processing, and the collective revenue cycle management (RCM) tasks required to ensure appropriate reimbursement for billable service are all critical to financial success. However, all carry an administrative burden and associated costs.
A key factor driving this increase is the payors’ expanding use of artificial intelligence (AI) in prior authorization determination and claim adjudication. To overcome the many challenges and bring more efficiency to the process, many providers are turning to AI for some of their most burdensome RCM tasks, like coding and claim processing, and the collective tasks required to ensure appropriate reimbursement for billable service.
Leveraging AI in their RCM, providers can keep pace and level the playing field. Practical AI applications will help:
- Intelligent translation of payor response to facilitate quick workflow pathways
- Streamline the key workflows – and the overall RCM process
- Reduce costs
- Enhance the patient experience
AI in RCM allows providers to stay competitive and elevate financial performance to the level that empowers superior patient care. However, leveraging embedded AI in RCM requires good data. Let’s consider why that is important.
From Data Complexity to AI Clarity
AI predictions are driven entirely by data. The quality of the data used in AI development and training as well as the quality of the data used to prompt a prediction are key to intelligent outcomes. Without good data, organizations cannot generate the AI models that move a business forward. Building effective AI models requires an understanding of the data landscape – and the capability to monitor performance and adjust AI-driven actions and workflow.
Preparing for AI demands purposeful data modeling and constant vigilance at every step of the process to ensure data integrity and valid results. Ensuring data accuracy early in the revenue cycle enables meaningful AI-driven decisions driving a timelier and more efficient workflow downstream. Dirty or poorly structured data leads to unintelligent AI and ineffective AI-driven outcomes.
RCM data can be incredibly complex; understanding what data to use and how to interpret it can mean the difference between powerfully predictive and terribly misleading information. Robust AI models built from the right data can determine if a claim is likely to be rejected because of incorrect or incomplete payor information or patient service benefits and can also lead to the automatic resolution of most issues. They can quickly enhance the probability of reimbursement and prioritize the remaining claims that require intervention. These claims are then directed to the best available team member for resolution.
Properly built AI delivers real, tangible value in the revenue cycle by removing friction from patient engagement and ordering physician experiences, reducing administrative burden, and accelerating claims processing. But how do you determine the real value of AI in RCM? It comes down to how performance, productivity, quality, and profitability are enhanced.
Maximizing the value of AI investments requires the use of AI modules that can be easily integrated into the RCM workflow architecture. Workflow integration also allows for AI models to be trained on RCM historic data directly, without interfaces or “bolt-on” frameworks that can be expensive to maintain.
Effective RCM leverages AI-driven process automation and provides user-configurable workflow automation by design. The architecture should be built to facilitate user-configurable workflow automation, and incorporate analytics-informed workflow recommendations. The ability to identify coverage and denial trends to direct workflow configuration adjustments is also important.
AI Saves Time, Reduces Cost
Healthcare executives looking to maximize reimbursement and keep the cost of collection low will want to explore how to better leverage data, AI, automation, and analytics across their RCM processes.
When looking to implement AI with a current or potential revenue cycle solution, keep these six factors in mind:
• Solution provider needs to have a deep understanding of healthcare data models and metrics specific to financial and operational workflow
• Look for AI that is embedded and leveraged throughout the revenue cycle
• Ability to scope and deliver business-critical metrics and indicators is key
• AI models should be customizable and reusable and integrate data from multiple sources
• Provider should be comfortable with various AI approaches and able to match them to specific needs (e.g., statistical, machine learning, natural language processing, and/or generative AI)
Embedded AI saves time and money and delivers better insights by removing friction for the patient and ordering physician, reducing administrative burden, and ultimately accelerating claims processing. Effectively using embedded AI requires understanding and commitment to accurate, well-structured data, expertise in various AI model types, and careful consideration of key criteria.
_____________________________________________________________
About Jeff Carmichael
Jeff Carmichael’s engineering leadership spans over 20 years and encompasses networking, security, and healthcare software and systems. He brings a career-long focus on data-driven insights and prediction through advanced data modeling across several industries. Prior to joining XiFin, Jeff led worldwide software development for the network and security division of LSI Corp. He has also held senior level leadership positions at several successful startups, and divisional leadership positions at Intel.