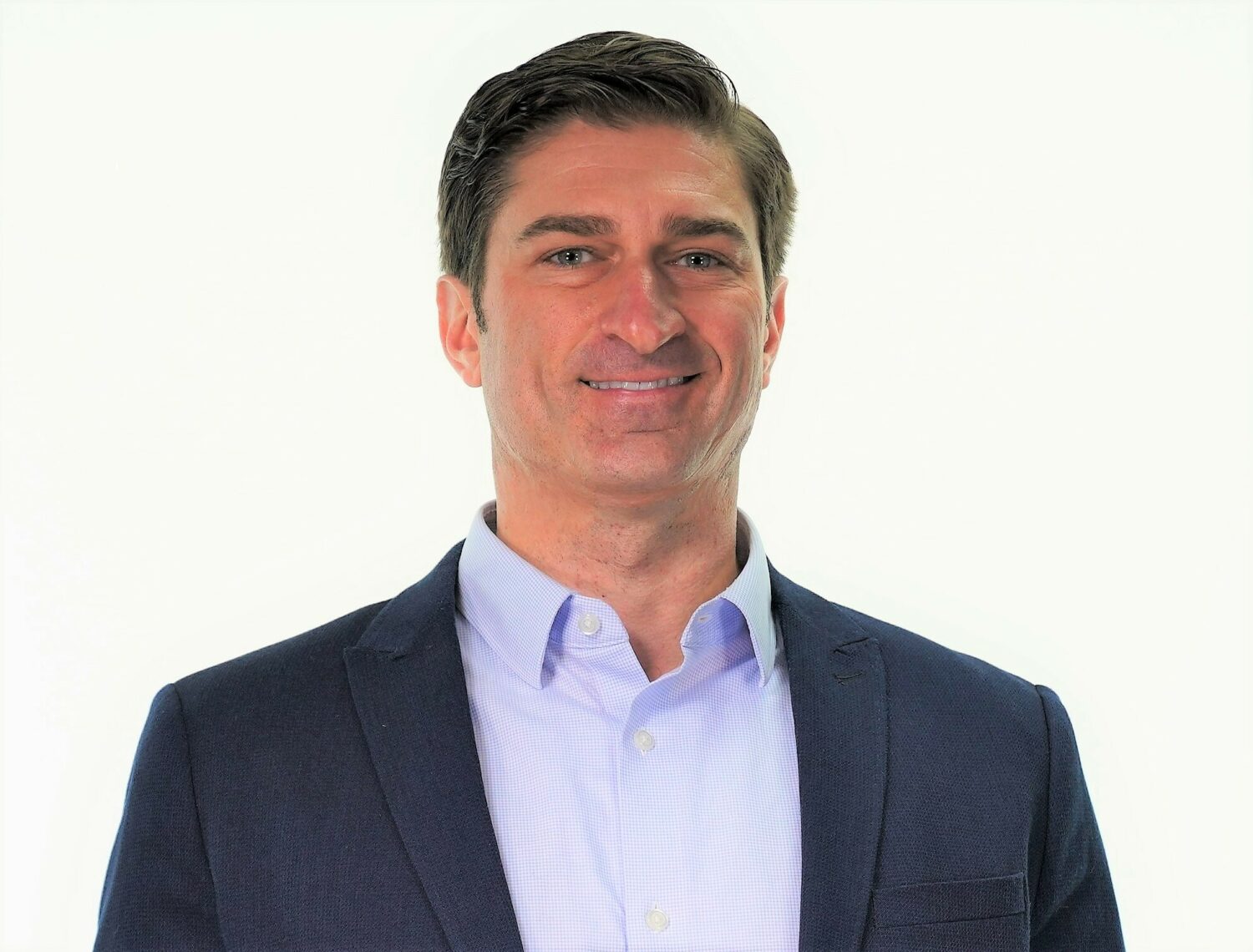
Signal detection — one of the most critical activities within pharmacovigilance (PV) — is the process of searching for and identifying safety signals from a variety of data sources. PV teams typically monitor safety signals for post-market products by reviewing scientific and medical literature, data obtained from clinical studies, and individual case safety reports (ICSRs).
ICSRs gather data supporting reporting adverse events (AEs), product problems, and customer complaints associated with regulated products. The four core elements of a valid ICSR include:
- An identifiable patient.
- An identifiable reporter.
- A suspect drug.
- An adverse event.
Suppose a disproportionate number of AEs for a product emerges. In that case, highly trained risk physicians or safety scientists analyze cases and other available evidence to confirm or reject a causal relationship between AEs and the suspect drug. This time-consuming and complex process requires several manual steps and depends on basic signal detection software that has been criticized by some as having a maturity gap.
Although ICSRs effectively indicate potential problems, ICSRs don’t come to PV signal teams instantly. Signal and risk physicians must sometimes process thousands of cases and reference outside sources of medical literature to make a determination. Another challenge? ICSRs don’t always provide enough detailed information for PV signal teams to draw a definitive conclusion about patient reactions to specific treatments. ICSRs are inherently delayed. Signal and risk physicians only gain visibility once patients or health professionals report an AE (not all AEs are reported).
PV signal teams must balance growing workloads with maintaining regulatory compliance. To help with that balance, life sciences organizations need to embrace operational efficiency upgrades like advanced cognitive computing to manage rising case volumes, increased signal noise, and antiquated manual tasks.
Advanced cognitive computing: The key to efficiency
Life sciences organizations failing to implement technology designed to improve efficiency and analyze data without overburdening safety teams will lag in the industry. Advanced cognitive computing capabilities, including machine learning (ML), boost operational performance, support greater compliance, and increase control by streamlining workflows, maximizing signal and risk physician output.
New innovation allows advanced cognitive computing to process data from a variety of disparate sources, accelerate the analysis of a case series — a group of case reports involving patients given similar treatment — and produce high-quality signal assessments. Advanced knowledge graphs, in particular, help PV signal teams focus and generate insights for target prioritization in drug discovery and post-marketing settings.
Advanced cognitive computing unlocks exciting opportunities for PV signal teams to increase their strategic contributions and catapult growth. In the coming years, more drug sponsors and contract research organizations (CROs) will use these technologies to drive faster time to insight, identify risk more quickly, and unlock new uses for existing medicines.
Embracing real-world data (RWD)
To advance patient safety, PV teams must leverage real-world data (RWD) and ICSR data. RWD — which reflects a broader group of patients — can systematically add additional valuable context to signals found in reported AE data sets. Drug developers gather RWD from:
- Electronic health records (EHRs): Digital patient charts featuring information about medical history, treatment plans, diagnoses, immunization dates, allergies, and more.
- Claims and billing activities: Data about healthcare services, population coverage, and patient prescribing patterns.
- Product and disease registries: Data collections defined by a particular condition, disease, or exposure.
- Patient-generated data: Information collected directly from a patient through mobile devices and wearables that provides real-time health status updates to medical teams.
- Social media: Posts made by patients on forums or social media platforms, including unsolicited, first-hand data about a treatment or drug.
RWD facilitates faster risk detection and gets closer to the patient, helping organizations avoid overreliance on ICSR. Not only can RWD add value to safety signals, RWD allows organizations to uncover correlations between drugs and benefits, opening the door to drug repurposing. For example, if researchers identify a correlation between a drug and a reduction in blood pressure for hypertension patients, they’ve unlocked a new way for their organization to help patients and drive revenue.
While the life sciences industry has been slower to adopt RWD (only 32% of life sciences organizations connect with RWD to drive drug development), regulators recognize the value of RWD sources and are leading the way in innovating signal detection approaches using RWD.
Take the Sentinel Initiative — a national electric system created by the FDA designed to answer questions about approved medical products like vaccines, drugs, and medical devices. The system generates computer programs for analyzing electronic healthcare data and studying relationships and patterns in EHRs and medical billing information.
—
Advanced cognitive computing and RWD will redefine the status quo for pharmacovigilance teams across the globe. Life sciences organizations must embrace these innovations to keep pace with growing safety signal volumes, streamlining the safety signal and analytics process, and becoming industry leaders benefiting humankind.
About Art Brown
Art Brown is the Director of Product Marketing, Data & Analytics at ArisGlobal. Art has 18 years of experience, primarily serving in health IT SaaS solutions, PBM/pharmacy services, and care delivery at major health systems and companies such as CVS Health. He applies his experience to help life sciences organizations understand the true value SaaS-based solutions can bring to their organizations and, ultimately, the patients they serve.